
Other examples of continuous data include temperature in a given space, time taken to complete a task, and length of a film. When continuous data is observed and collected in a dataset, statistical procedures can be used on the determine the mean, mode, range, standard deviation, and other statistical characteristics of the data. For example, someone's height would be considered continuous data.
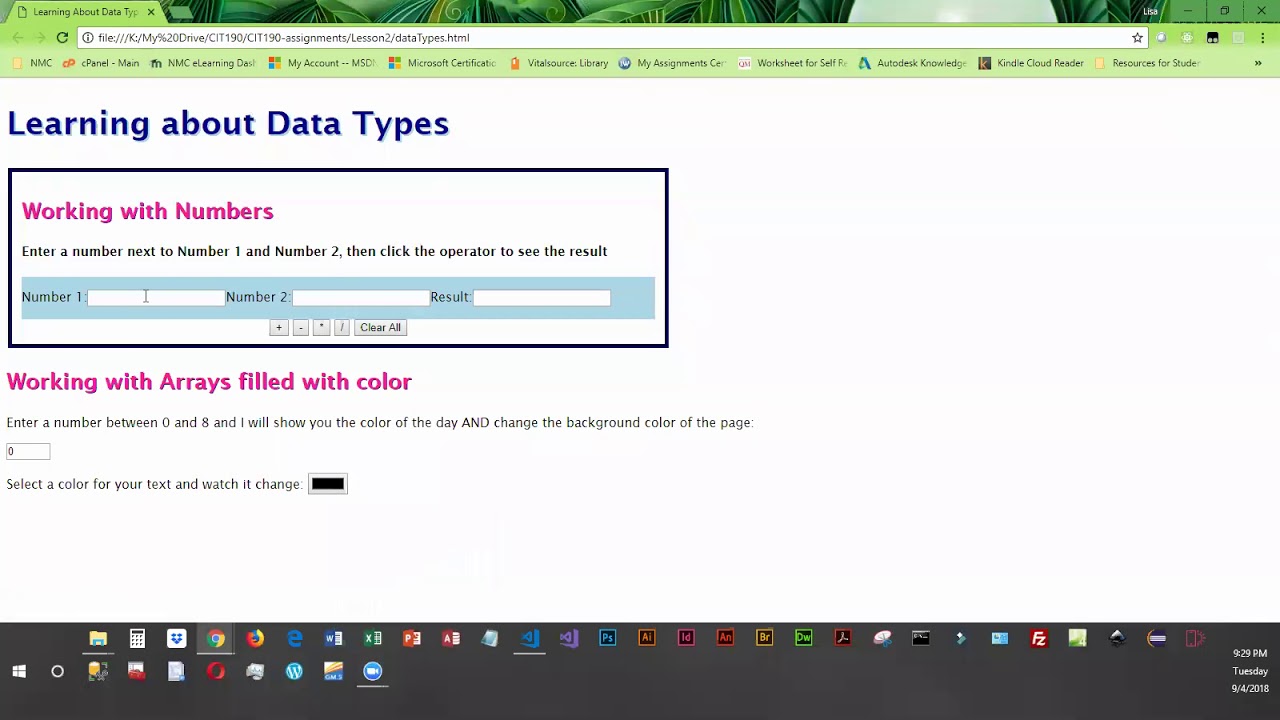
Other examples of discrete data include the number of students in a classroom, the number of computers in a given space, and the number of light fixtures in a commercial property.Ĭontinuous data are data that have quantitative (numeric) value but are measured instead of counted. With this longitudinal view, you may ask the average cars parked on campus over a given temporal period (day, week, etc.). This aggregate number can be subdivided based on a categorical feature - there are 10 blue cars, 30 red cars, and 10 green cars. You can run more advanced statistical tests on discrete data if you observe the data over time. This data type can be described statistically in aggregate - as a single number, for example, there may be 50 cars parked on campus. For example, the total number of cars parked on campus would be considered discrete data. Qualitative data can be further divided into discrete data, continuous interval data, and continuous ratio data.ĭiscrete data are data that has quantitative (numeric) value and can be counted, not measured. For example, someone's shoe size would be considered qualitative information. Qualitative data are data that have numerical value and can be processed using statistical methods. Other examples of ordinal data include academic grades (A,B,C,D,F), education degree level (Bachelor's, Master's, Doctoral), and satisfaction rating (extremely dissatisfied, dissatisfied, neutral, satisfied, extremely satisfied). This data type can be described statistically in terms of frequency. For example, someone's final place in a race would be considered ordinal data: first, second, third, etc. Ordinal data are data that can be categorized and has a natural ordering but it has no quantitative (numeric) value. Other examples of nominal data include hair color, religion, sexual orientation, college major, and preferred cell phone carrier. For example, someone's nationality would be considered nominal data: American, British, French, etc. Nominal data are data that represent categories and have no quantitative (numeric) value or natural ordering.
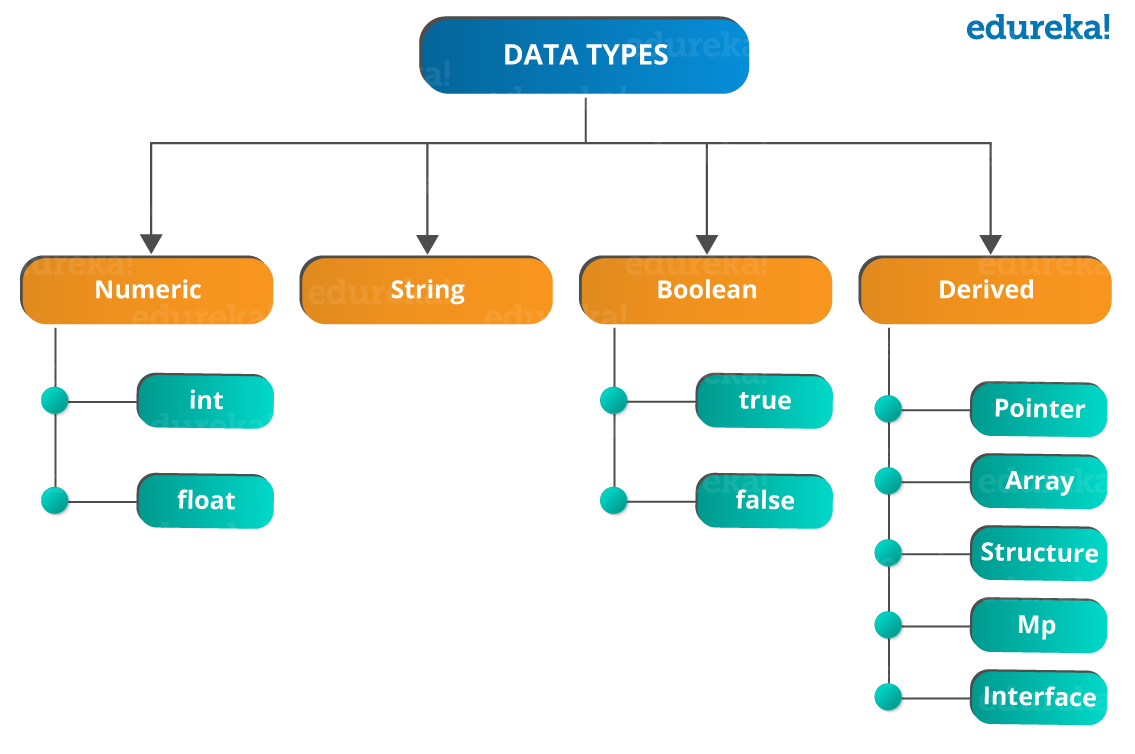
Nominal data and ordinal data are the two qualitative data types. Though mathematical calculations cannot be used on qualitative data, descriptive statistics such as frequency can describe qualitative data. For example, someone's affiliation to the university (student, staff, faculty) would be considered qualitative information. Qualitative data are data that have no numerical value but rather represent categories or concepts. Since data can take various forms, understanding the differences and intersections of qualitative and quantitative data can help you produce data-informed insights. Qualitative and Quantitative Data Explained
